Written by:
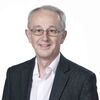
VP Discovery Biology, Discovery Sciences, R&D
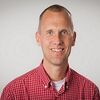
Head of Target Science, Research and Early Development, Respiratory and Immunology, BioPharmaceuticals R&D
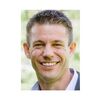
Head of Translational Science and Experimental Medicine, Research and Early Development, Cardiovascular, Renal and Metabolism, BioPharmaceuticals R&D
Target identification lies at the heart of modern drug discovery. On paper, the process sounds simple - find a biological target that plays a role in disease, then find a therapeutic that interacts with it - yet this belies the complexity of the task.
The challenge of discovering and validating targets is reflected in the failure rate of drug candidates in the clinic, where promising treatments fail to show efficacy even in relatively late stage trials. The reason for this failure is usually that the underlying hypothesis - that this drug activates or inhibits a target and modulates the disease in a particular way in a particular patient population - turns out to be wrong.
While our success rate of pipeline molecules advancing from pre-clinical investigation to completion of Phase III clinical trials is higher than industry averages, we endeavour to do better. For this reason, over the past few years we have invested in multiple technologies to help improve target discovery.
More or better targets? From genotype to phenotype
In the past, most of our drug targets have been found by combing the published scientific literature for insights into molecular pathways or genetic variants linked to disease. We’re now aiming to get ahead of the curve by focusing on the identification of original novel targets through our recent investments in genomics, functional genomics, and machine learning and artificial intelligence (ML/AI).
The journey to discovering better targets starts with building a deep understanding of biology. Increasingly, this comes from genomic insights, whether from patients and public biobanks or from tissue and tumour samples, aiming to identify genetic alterations underpinning disease.
Through our Centre for Genomics Research, we’re aiming to analyse 2 million genomes by 2026, drawn from diverse populations and covering a wide range of diseases and clinical trials.
However, this approach isn’t about mining genomic data to generate ever-longer lists of possible genes as targets for drug screening. We don’t need more targets, we need better ones, not just to reduce the failure rate in drug development but also in discovery when we go into cellular and animal models of disease.
While genomic data can reveal hints about important genes and pathways involved in health and disease, it can’t provide the full picture of what’s going on within cells, tissues, organs or the whole body.
To do this, we’re taking a functional genomics approach to search for novel targets, both internally and through collaborations, such as the AstraZeneca Functional Genomics Centre with Cancer Research UK, and partnerships with Horizon Discovery and the Innovative Genomics Institute.
We’re using large-scale CRISPR screens to systematically delete (CRISPRn), upregulate (CRISPRa) or downregulate (CRISPRi) every gene in the genome, looking for phenotypic outcomes that accurately mimic the desired effect of a drug.
Ultimately, functional genomics allows us to more fully understand the relationship between genotype and phenotype, to build disease-relevant models and assays for CRISPR-based or other types of screening. It underpins the discovery and validation of novel targets, enables us to elucidate mechanism of action and the biological pathways affected in disease, and can also highlight potential targets for synthetic lethal approaches.
Applying AI to generate insights
Advances in technology and falling costs now make it possible to carry out multiomic characterisation of tissues from thousands of patients - including genomic, transcriptomic, proteomic, metabolomic and lipidomic analysis, together with multimodal imaging and detailed clinical data - in order to build up a phenotypic ‘fingerprint’ of the disease state and patient population.
From here, not only can we uncover pathways and targets that are responsible for driving disease, but we can spot shared mechanisms underpinning conditions with complex, often overlapping phenotypes.
Many diseases like liver, kidney and heart disease are driven by common mechanisms such as inflammation, yet we try and treat them all as separate entities. We’ve been hampered in the past by approaching the science in a very siloed way, just focusing on one specific organ or disease, when in fact these conditions are linked by common biology.
Analysing multiple complex datasets in this way is only possible thanks to advances in computing. In recent years we’ve seen the development of powerful ML/AI tools based on knowledge graphs, which capture detailed information about objects or concepts - such as genes, drugs, diseases or molecular pathways - and the relationships between them.
Exploring this interconnected data space can generate novel insights and identify potential drug targets, as well as revealing opportunities where existing drugs might be repurposed. And although the usual caveat of data quality still applies (‘garbage in, garbage out’), this approach is bearing fruit.
For example, our collaboration with BenevolentAI is already enabling us to identify novel targets in chronic kidney disease through their knowledge graph and our wealth of data. We’ve now started our first drug discovery project resulting from this collaboration based on a previously unexplored and unexpected target involved in podocyte function in the kidney, which we validated in various preclinical models, with a second project coming soon.
The challenge of target validation
However it has been identified, any potential target must be investigated to prove that it is relevant to the disease and that modulating it will have the desired outcome. And that depends on having relevant, physiologically realistic disease models and phenotypic assays.
Today we have a range of tools and methods for generating models available to us, such as genome engineering and advanced tissue culture techniques, to create in vivo models with species such as zebrafish and mice or develop next-generation in vitro models based on primary cells, induced pluripotent stem cells, organoids, co-cultures, and ‘lab on a chip’ microfluidic technology.
As an example, if we are to find more effective ways to halt the progression of lung disease or even reverse it and repair fibrotic damage, we must be able to model all parts of the lung, including the small airway epithelium, alveoli, fibroblasts and immune cells, and combine them in both structural and functional ways to mimic the disease phenotype.
Staying on target
Improving target identification and validation through the integration of genomics, functional genomics and ML/AI has the potential to transform drug development over the coming years. Each approach individually will generate lists of targets, but the true power lies in identifying synergies and shared insights that emerge across all three.
Currently around 10 per cent of the new projects in our portfolio are derived from these methods, and we’re aiming to make it a quarter by 2023. In five years we would like to be in a place where half of our projects arise from in-house or collaborative target discovery, with physiologically-relevant validation for all of them.
At the same time as our tools and technologies for target discovery and validation are improving, we’re also seeing a dramatic expansion in therapeutic modalities, opening up opportunities to target biological pathways in completely new ways. We have a whole toolbox of modalities, including cell and gene therapies, novel antibodies and other biologics, peptides and nucleic acids, so we can expand the druggable space and address any target we find.
We’re working towards an aim where no drug candidate fails because it doesn’t work in a relevant model of disease or patient population. If we can find better targets and deliver medicines that are more likely to succeed in the clinic, we’ll speed up drug discovery, and ultimately improve the quality of therapies and outcomes for patients.
If you’re interested in learning more, listen to the latest episode of the Genetics Unzipped podcast, where Steve Rees (VP Discovery Biology, Discovery Sciences, R&D, AstraZeneca), David Goldstein (Director, Institute for Genomic Medicine, Columbia University Medical Center, New York, US) and Dave Michalovich (VP Precision Medicine, BenevolentAI) talk about how we can use the power of genomics, functional genomics and AI to discover new targets and make better medicines.