Written by:
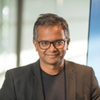
Executive Director and Head of Emerging Innovations, Discovery Sciences, AstraZeneca
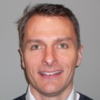
Senior Director of Emerging Innovations and Indication Discovery, Discovery Sciences, AstraZeneca
The pace of change in healthcare is moving more rapidly than ever before. By harnessing new data and technologies, we are creating a dynamic and evolving landscape within drug discovery. To maximise the delivery of potential new medicines, we are using data driven methods to turn traditional drug discovery methods upside down.
What is drug repurposing?
Drug repurposing, sometimes called repositioning, is finding new uses for existing medicines. This involves taking a drug compound that has initially been tested in one disease and exploring its use to treat a different disease. At AstraZeneca, we are using our knowledge gained in this area of science to potentially speed the time to get new medicines to patients.
Starting in 2015 through a collaboration with Joel Dudley and the Icahn School of Medicine at Mount Sinai, we developed an unbiased approach to drug repurposing using billions of data points.
Using data driven methods to inform drug repurposing
Diseases result in different gene expression in the body. The specific genes that are affected and whether their expression increases or decreases depends on the type of disease. We can measure this change in gene expression by generating multi-omic signatures which are unique to individual types of diseases. We can also produce a similar signature – called a drug signature – when medicines interact with cells in the body resulting in changes in gene expression.
Transcriptomic mapping is a digital-based approach which compares drug signatures to disease signatures and generates a connectivity score to find complimentary matches between the two. Using digital technologies and artificial intelligence on our in-house databases along with external data sources, we can compare drug signatures to disease signatures at the molecular level and find “matches”. The complimentary matches indicate potential medicines that could be repurposed for certain diseases.
Though it sounds simple, the technology used to perform these analyses is quite sophisticated since disease profiles often contain extraneous variables that must be accounted for. For example, a patient may have asthma but at the same time, also have an infection. How can researchers be sure that the disease signature they observe is due to the asthma alone and is not influenced by the infection?
In short, algorithms and data analytics are employed to clean the synthetic signature of the patient data before running any connectivity analyses. This crucial first step improves the accuracy of this approach.
Learn more about how this technology works in the animation below
New research findings in idiopathic pulmonary fibrosis (IPF)
Recent research in the American Journal of Respiratory and Critical Care Medicine conducted in collaboration with Yale University, National Jewish Hospital, and Icahn School of Medicine at Mount Sinai used this method as a starting point to identify complementary gene expression profiles induced by idiopathic pulmonary fibrosis (IPF) and saracatinib, a candidate drug originally developed to potentially treat cancer.
Once saracatinib and IPF were found as a match using the computational approaches, researchers conducted laboratory experiments using preclinical models of IPF to test the effectiveness of the candidate drug. Saracatinib was shown to work as well or better than available treatments in countering fibrosis in cultured human lung cells and fibrotic lung slices obtained from IPF patients. Now, saracatinib is being tested in patients with IPF as part of a clinical trial conducted by the academic investigators and funded by the National Institutes of Health (NIH).
A bright future ahead
Novel insights such as this showcase the possibilities for using this approach in drug discovery. From discovering new indications for medicines, to finding novel and more effective drug combinations, optimised data driven methodologies have the potential to help transform the way we discover and develop new medicines for the patients who need them most.
Within AstraZeneca, our efforts to optimise transcriptomic mapping continue as we build on these data driven methodologies by leading in the development of omics-mapping and AI-based graph representation learning of biomedical knowledge graphs with the aim to predict novel links between candidate drug compounds and diseases.